Customers are the greatest source of learning for a business. If a customer is unhappy or happy, there’s something about the company, product, or customer service experience that made them that way. With millions of calls recorded, contact centers contain valuable information about a company’s customers. Unlike manual investigation, Sentiment Analysis tools can extract this information from recordings of customer conversations in an efficient and fast way. In this article, we discuss sentiment analysis in a contact center and answer several important questions about this topic, starting with what is sentiment analysis, how does it work and how to take advantage of it?
What is sentiment analysis in a contact center?
Contact centers are increasingly offering native sentiment analysis tools that analyze conversations (voice and chat) between customers and agents. Among these contact centers, we cite AWS Connect and Genesys Cloud which offer this functionality. Most importantly, this feature can be activated in a few clicks, configured quickly, and requires no prior knowledge of coding, machine learning, or underlying algorithms. Subsequently, all conversations are analyzed in order to deduce the feelings of the interlocutors, which are the agent and the customer in a contact center.
By definition, sentiment analysis is an automatic text classification tool to infer, as the name suggests, sentiment. Basically, the inferred sentiment can be classified into three categories: positive, negative, or neutral sentiment. In this case, it is the dominant feeling or the polarity of the text. Sentiment analysis can also go beyond polarity and detect more precise feelings such as anger, disgust, fear, surprise… Figures 1 and 2 show the sentiment analysis interface of AWS Connect and Genesys Cloud respectively. AWS Connect points the sentiment score via either a smiley, neutral, or sad face for each reply in the conversation between the customer and the agent, while Genesys cloud uses the thumbs up or thumbs down emoticon placed on the record.
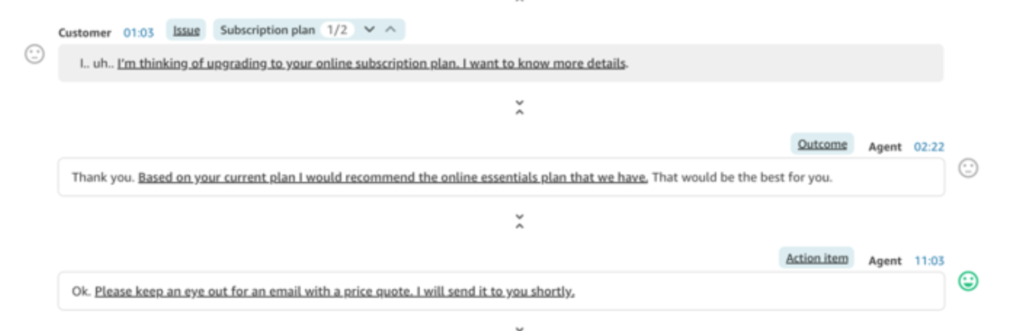
Figure 1: Ex. sentiment analysis from AWS Connect

Figure 2: Ex. Genesys Cloud Sentiment Analysis
How does it work?
Any sentiment analysis tool is based on two main pillars: natural language processing algorithms and machine learning algorithms. Sentiment analysis systems are mainly based on the analysis of textual content. As a first step, call recordings must be converted into text and more precisely into conversation between interlocutors. This step is also known as the transcription step. Keywords and key phrases are then extracted from the text for each replica. Via “machine learning”, an individual score is assigned to each replica. Finally, a general score is attributed to the conversation which is in general the average of the individual scores. To summarize, the steps are as follows:
- Transcribe voice recording to agent and customer chat text
- Extract phrases and keywords
- Assign a sentiment score for each line
- Combine the individual scores to get a conversation score
To be able to assign a sentiment score in the third step, the sentiment analysis system uses a “sentiment dictionary”. This is a very large collection of adjectives (ex: good, bad, wonderful, awful, happy, sad) and phrases (ex: I like, I don’t like, it’s great, it doesn’t suit me, it’s wonderful, it’s awful) that have been assigned a score by hand. So, for example, phrases that contain or sound like “Like” will be classified as “positive” while phrases containing “I don’t like” will be classified as “negative.”
Contrary to what some might think, sentiment analysis is not based on pitch or loudness. However, we can measure the loudness of the speech of the customer and the agent and use this information to complete the sentiment analysis. For example, a high sound intensity accompanied by a negative feeling can be a strong indicator of dissatisfaction. However, you will have to read or hear this part of the conversation to try to understand and solve the problem.
How to benefit from sentiment analysis in a Contact Center?
There are certainly several advantages to using sentiment analysis tools in a contact center. For starters, managers gain in efficiency and a huge amount of time because they no longer need to manually analyze sentiment and listen in on every conversation.
As Bill Gates said, “Your most unhappy customers are your greatest source of learning”. So, a first course of action is to spot conversations with a high level of negative feelings. Then, we can use these conversations to better coach agents and provide new guidelines in favor of the customer. We can also use these conversations to offer personalized service to the customer and better meet their needs. In addition to this, we can identify the agents with the highest levels of positive emotions and positive conversations to reward them, encourage them and make them ambassadors. Finally, it can be interesting to visualize sentiment trends and correlations such as:
- Sentiment trends for an agent, for a team, for a client
- Trends in sentiment based on a product, service, or marketing campaign
- The correlation between the customer’s feeling and the duration of the call, the duration of silence (non-talk time).
Based on this information, we are able to make relevant decisions to improve the feeling of both the customer and the agent.
Conclusion
In conclusion, sentiment analysis in a contact center brings unprecedented added value to the brand, helping it to obtain important information on what makes the customer happy or unhappy. This functionality, which was then expensive, complex and difficult to implement a few years ago, is now available, affordable, and simple to implement. To take advantage of it and to find out more, contact us to discuss these subjects for which Activeo has in-depth expertise, and we will present you with use cases and feedback.